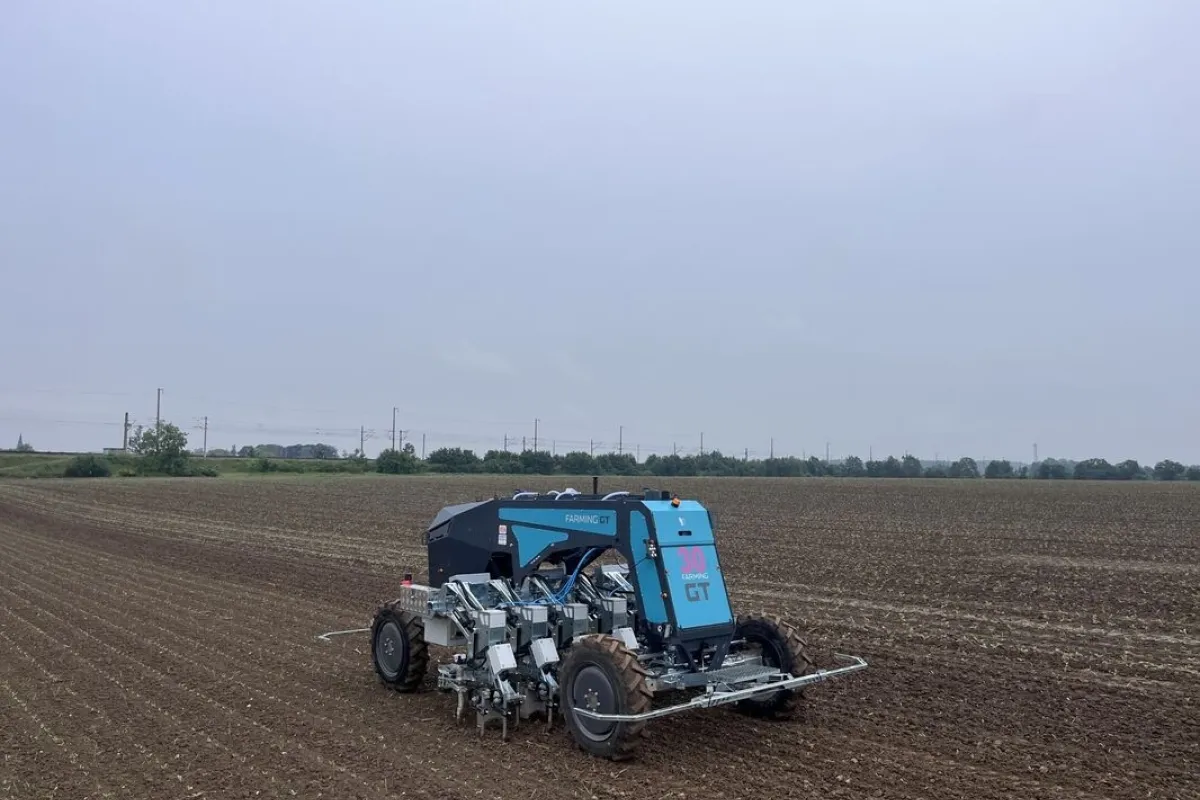
Overview
Through this service, we offer customers the ability to validate and augment the data collected during the different testing phases. Examples of operations that this service can provide include quality checks (e.g., presence of all datastreams, presence of drop-outs, technical quality such as focus or exposure of camera images, agronomic significance of data); preparation for performance evaluation (e.g., identification of the images containing selected visual markers or specific plants, association between images from the system under test and the ground truth); data processing (e.g., transcoding) and augmentation (e.g., generation and incorporation of metadata). Data labelling (e.g., to enable training of AI models) is not included, as it is the object of a separate service (S00291).
More about the service
Such activities can require a very wide range of specialised expertise, comprising disparate items such as know-how about what can be expected by a given type of sensor in a specific experimental condition (e.g., to detect if the technical quality of sensor streams is subpar), agronomic know-how (e.g., to identify which of the plants appearing in a video stream correspond in their growth stage or disease status to the use case targeted by the customer), or the capability of writing custom transcoding software (e.g., to make the data compatible with a given software package).
This service provides the customer with access to an expert group of engineers and agronomists that possess, collectively, the whole range of capabilities to perform all of the above data operations and more. When needed, the service also provides the processing resources required for the execution of complex data processing tasks.
The preparation phase of the service involves one or more interviews where the customer shares with AgrifoodTEF information about the data to be processed and the goals that they need to attain through such processing. Additional information (such as the technical specifications of the (sub)systems used to generate the data) may be requested to enable the activities of AgrifoodTEF; any confidential information will be shared under NDA.
The preliminary exchange of information is followed by the transmission to AgrifoodTEF of the data to be processed. Transmission can occur, for instance, by providing AgrifoodTEF with either a copy of the data or access to a repository where the data are stored. At the end of the service, the customer receives the processed data and a report detailing all issues detected in the original data (if any) and the impact that they had on the augmented data provided by the service.
To enhance the quality of the hyperspectral data, we apply opportune noise reduction methods to the data. Additionally, let us assume the customer data were all collected in a single run at 9:00 AM. In this case, we would diversify the brightness of RGB images in post-processing to obtain a more heterogeneous and rich set that resembles different lighting conditions.