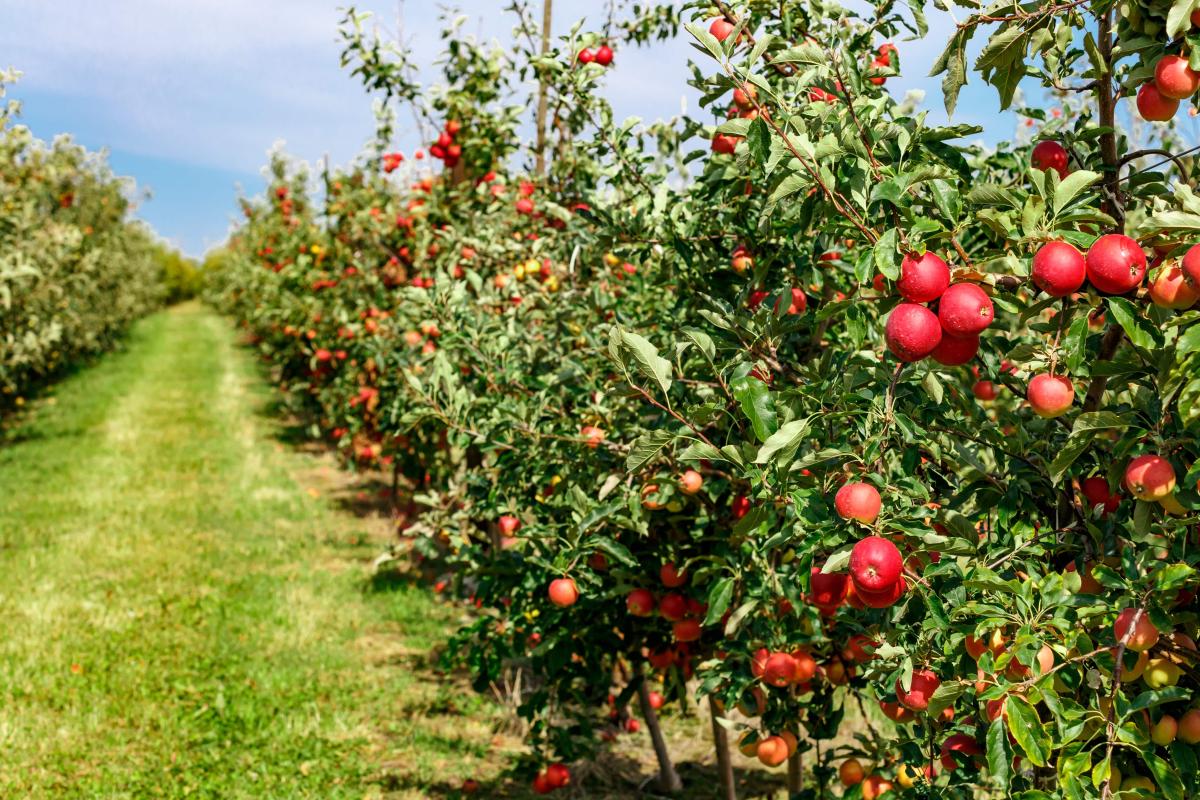
Overview
Given a dataset intended to be used in AI or robotics applications for the agrifood sector, a quality analysis will be performed to search for issues in the provided data. Thus, the customer can ensure their data is reliable and suitable for use in this kind of application. The dataset could be an input or an output of an AI process. For example, it could be data to train a predictive system or the output of that predictive system (which allows detecting prediction problems, like predictions out of range or not following the expected output distribution). The kind of issues to search for range from missing values to problems related to data out of distribution. As a result of this service, the client will receive a report with the conclusions and recommendations on how to improve the data, if needed.
More about the service
They might have concerns about data quality but lack the expertise or tools to properly evaluate it.After using the service, customers receive a comprehensive analysis that identifies potential problems in their data, such as missing or incomplete entries, outliers, or values that deviate from expected patterns. This is particularly valuable for organisations that are developing or implementing AI solutions, as poor data quality can lead to unreliable model predictions or unsafe robotic operations in agricultural settings.
For example, a company using AI for crop yield prediction would want to ensure their historical farming data is complete and consistent before training their models. The service would help them identify any gaps in sensor readings, abnormal weather measurements, or inconsistent formatting that could affect their predictions. Similarly, a business using robots for fruit picking would benefit from knowing if their training data contains edge cases or unusual scenarios that might cause the robot to make mistakes during operation.By identifying these issues early, the service helps customers avoid costly mistakes, improve the reliability of their AI systems, and make more informed decisions about data collection and preprocessing.
This is especially crucial in the agrifood sector, where AI and robotics decisions can have significant impacts on crop yields, food safety, and operational efficiency. The detailed report provided helps customers understand exactly what needs to be fixed in their data before proceeding with their AI or robotics implementations.
The service can be delivered remotely since it involves computational analysis of digital data.
The execution time typically ranges from 1-2 weeks, depending on dataset size and complexity.
The customer receives a detailed technical report documenting:
- Data quality issues found.
- Statistical analysis results.
- Recommendations for data improvement.
There are no seasonal restrictions or location requirements. The service can be performed year-round for customers worldwide, if they can securely share their data electronically.
Key customer requirements:
- Provide dataset access.
- Share context about intended AI/robotics use.
- Specify quality parameters of interest.
- Available for clarification questions during analysis.
Customers can select focus areas like completeness, consistency, accuracy, or timeliness of data.The analysis can be tailored for different agricultural applications such as crop monitoring, harvesting robots, or yield prediction systems.
This includes customising thresholds for outlier detection and adapting validation rules to specific crop types or farming conditions.Key limitations:
- Analysis limited to structured digital data.
- Requires a minimum dataset size for meaningful statistical analysis.
- Cannot fix identified data quality issues (only provides recommendations).
- Processing time increases with dataset complexity.
- May require domain expertise input for specialised agricultural contexts.
Customers should provide clear documentation about their data collection methods and intended use case to enable proper customisation of the analysis parameters.