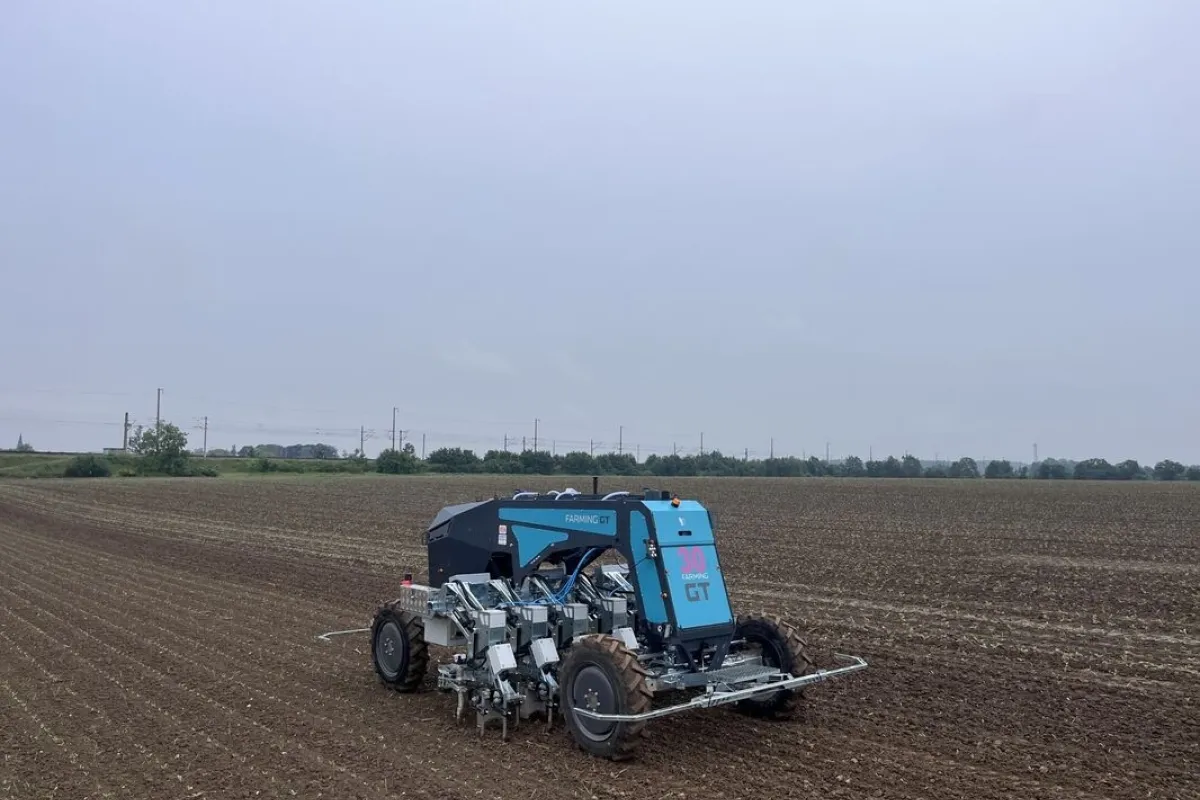
Overview
This service consists of deploying a modular sensor payload (equipped with Lidar, inertial motion units, GNSS, Radar, stereo cameras, and ultra-wideband technology) to agricultural environments such as vineyards and orchards to collect and annotate high-fidelity datasets tailored to customer needs. Data are systematically labelled and can be delivered to agrifoodTEF customers as structured datasets for AI-driven agricultural solutions.
More about the service
AgrifoodTEF customers (e.g., agricultural robotics/AI developers, precision farming companies, or researchers) often lack access to high-quality, annotated, real-world datasets from dynamic agricultural environments (e.g., vineyards, orchards).
Without this, they struggle to:
- Build robust AI systems and use cases (e.g., for crop monitoring, robotic harvesting, or autonomous navigation).
- Validate system performance under realistic, variable conditions (e.g., uneven terrain, changing light/weather).
- Benchmark their solutions against industry standards or competitors.
Solution (After): By deploying the sensor payload to collect and label data in real-world settings, the service enables customers to:
1. Build AI models effectively with diverse, context-rich datasets (e.g., labelled images of fruit clusters, 3D terrain maps).
2. Validate system reliability by testing solutions against benchmarks (e.g., "After using the service, a robotic harvester’s error rate in detecting ripe fruit drops from 25% to 8% in cluttered orchard conditions").
3. Identify and fix weaknesses (e.g., a navigation algorithm fails in GNSS-denied rows; the service provides UWB/IMU data to refine localisation).
4. Compare performance against competitors using standardised metrics (e.g., "Our weeding robot achieves 95% accuracy vs. the industry average of 82%").
This service can be delivered as an initial step prior to other services.
1. How is the service delivered?
Deployment Model:- On-site execution:
A sensor-equipped payload (modular, vehicle/robot-mounted) is deployed to the customer’s agricultural site (e.g., vineyard, orchard) or partner test farms.
- Repetitions: Data collection can be performed as a single campaign or recurring sessions (e.g., seasonal cycles, growth stages) to capture variability (e.g., flowering vs. harvest periods).
2. When can the service be delivered?
- Scheduling: Requires advance booking (e.g., 4–8 weeks) for payload deployment and site preparation.
3. How long does execution take?
- On-site deployment: 1–2 weeks per campaign (adjustable based on farm size and data density).
- Post-processing: Additional 2–3 weeks for labelling and report generation.
- Total timeline: ~4–8 weeks from deployment to delivery.
Location & Customer Requirements
4. Where is the service executed? - Customer’s own site: Farms, orchards, or vineyards.
- Partner test farms: Pre-approved agrifoodTEF satellite nodes (e.g., standardised test fields for benchmarking).
5. Customer location requirements:
- Regional focus: Prioritises regions with agrifoodTEF infrastructure (e.g., EU initially).
- Global access: Possible via partnerships with local nodes (additional logistics fees may apply).
Customer Deliverables
6. What does the customer receive?
Outputs:- Raw & labelled datasets: Structured, timestamped sensor data (Lidar point clouds, stereo camera images, GNSS/IMU trajectories) in AI-ready formats (e.g., ROS bags, COCO JSON).
- Documentation: Metadata (sensor specs, environmental conditions), annotation guidelines, and compliance certificates.
- Summary report: Actionable insights (e.g., “System struggles in low-light conditions; recommend augmenting training with twilight radar data”).
Customer Obligations
7. What must the customer provide?
- Site access: Safe, operational fields for deployment (e.g., cleared rows for robot/sensor mobility).
- Use case specifications:
- Target metrics (e.g., “Collect data on apple cluster density in rows 5–10”).
- AI/system requirements (e.g., “Annotate fruit ripeness labels for YOLO training”).
- Ethical/legal compliance: Permissions for data collection (if required).
- Customers can select specific sensors from a predefined list of candidate sensors for the payload (e.g., prioritise Lidar + stereo cameras for 3D mapping, disable GNSS for indoor testing).
Data Annotation:
- Choose annotation formats from a predefined list (e.g., bounding boxes, semantic segmentation masks) compatible with their AI pipelines.
Temporal/Seasonal Flexibility:
- Schedule recurring campaigns (e.g., weekly data collection during harvest season, multi-year phenological studies).
Seasonal restrictions:
- Aligns with vegetation periods (e.g., no frost/rain for certain sensors; optimal timing for crop-specific data like fruit maturity in orchards).
- Example: Vineyard data collection restricted to the growing season (April–October in EU climates).
What Customers Should Know in Advance
- Site Preparation: Customers must provide field maps, irrigation schedules, and safety protocols.
- Data Ownership: Raw data is shared with the customer, but agrifoodTEF may use aggregated, anonymised data for internal R&D.
- Cost Implications:
- Recurring campaigns or ultra-high-resolution sensors incur higher fees.
- Post-processing of complex annotations (e.g., 3D point cloud labelling) billed separately