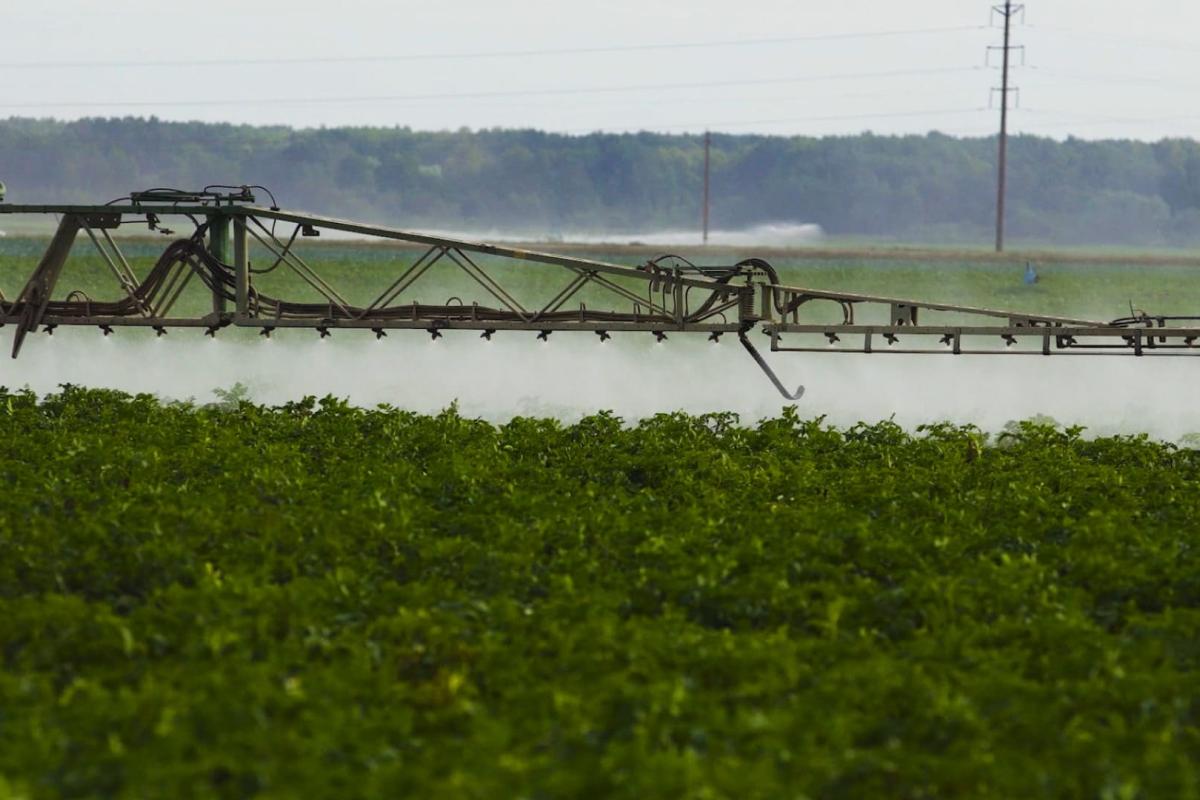
Overview
We offer experimentation fields for arable crops, horticulture crops, and greenhouse facilities. These environments can be complemented with agronomic validation services, sample laboratory analysis or crop characterisation if required.
All combined serve as testbeds for data acquisition throughout the crops’ growth cycle, providing the infrastructure and expertise to support companies in the testing, validation and improvement of their technological solutions in real agricultural conditions.
This includes:
- AI-based solutions, such as training and calibration predictive models and algorithms for agronomy applications, including automatic weed detection, biomass and yield estimation, phenological characterisation, or pest and disease monitoring.
- Robotic systems and machinery that require physical testing, such as autonomous vehicles, precision spraying equipment, harvesting robots, or treatment platforms.
More about the service
It enables the adjustment and adaptation of models, algorithms or systems to specific environments or real-world variability, such as different soil types, weather conditions, crop development stages, pest or disease presence, and diverse management practices. This provides a robust basis for improving performance, accuracy, and reliability.
• Before using this service: Technology may work in a controlled setting, but lack calibration for a specific real-field variability or realistic agronomic conditions.
• After the service: Companies receive comprehensive datasets and documentation, including field imagery, crop characterisation, field measurements, laboratory analyses and environmental parameters, enabling to fine-tune their solution for its optimal performance.
To illustrate:
• An AI-model algorithm developed to identify phenological stages in soybeans can be adapted and validated for maize using real-time drone imagery combined with crop-specific data collected throughout the whole growth cycle.
• A robotic platform for fungal control in vegetables can be evaluated through comparative field trials, assessing its effectiveness against conventional and organic treatments, under specific cropping conditions, supported by agronomic measurements and laboratory analysis.
• Design and planning of agronomic field trials (randomised blocks, number of replications, etc.).
• Implementation of the technology to test under predefined protocols and conditions.
• Comparative treatments (e.g. solution treatment vs conventional, organic, and control).
• In-field sampling and laboratory analysis for gathering relevant agronomic parameters (e.g. yield, biomass, disease observations, or nutritional composition such as water, nitrogen, carbon content, or any other physiological characterisation).
• Collection of environmental and contextual data (weather, soil, crop status).
Output deliverables include full datasets, detailed reports with interpreted results adapted to the customer’s needs, and other tailored documentation for the use case.
The duration of the service depends on the specific crop and its growth cycle, typically spanning several months.
Customisation options may include:
• Crop selection: cereals or other arable crops, vegetables or other horticulture crops, subject to seasonal and operational availability.
• Experimental setup: control treatments, organic vs. conventional management, or tailored layouts (e.g. randomised blocks, split plots), planting configurations, or sampling strategies.
• Measured parameters: plant health indicators, disease incidence, yield, biomass, AI model training outputs, etc.
• Environment type: open-field plots, greenhouse facilities, or precision agriculture-designed setups.
Limitations:
• While adjustments to crop species, varieties, or planting layouts can sometimes be accommodated, these must align with operational constraints and be planned well in advance. The feasibility of such customisations is subject to the seasonal calendar and the biological cycle of each crop.
Customers should communicate their testing requirements early to ensure the service meets their objectives. Any limitations regarding the feasibility of customisations will be discussed during the planning phase.